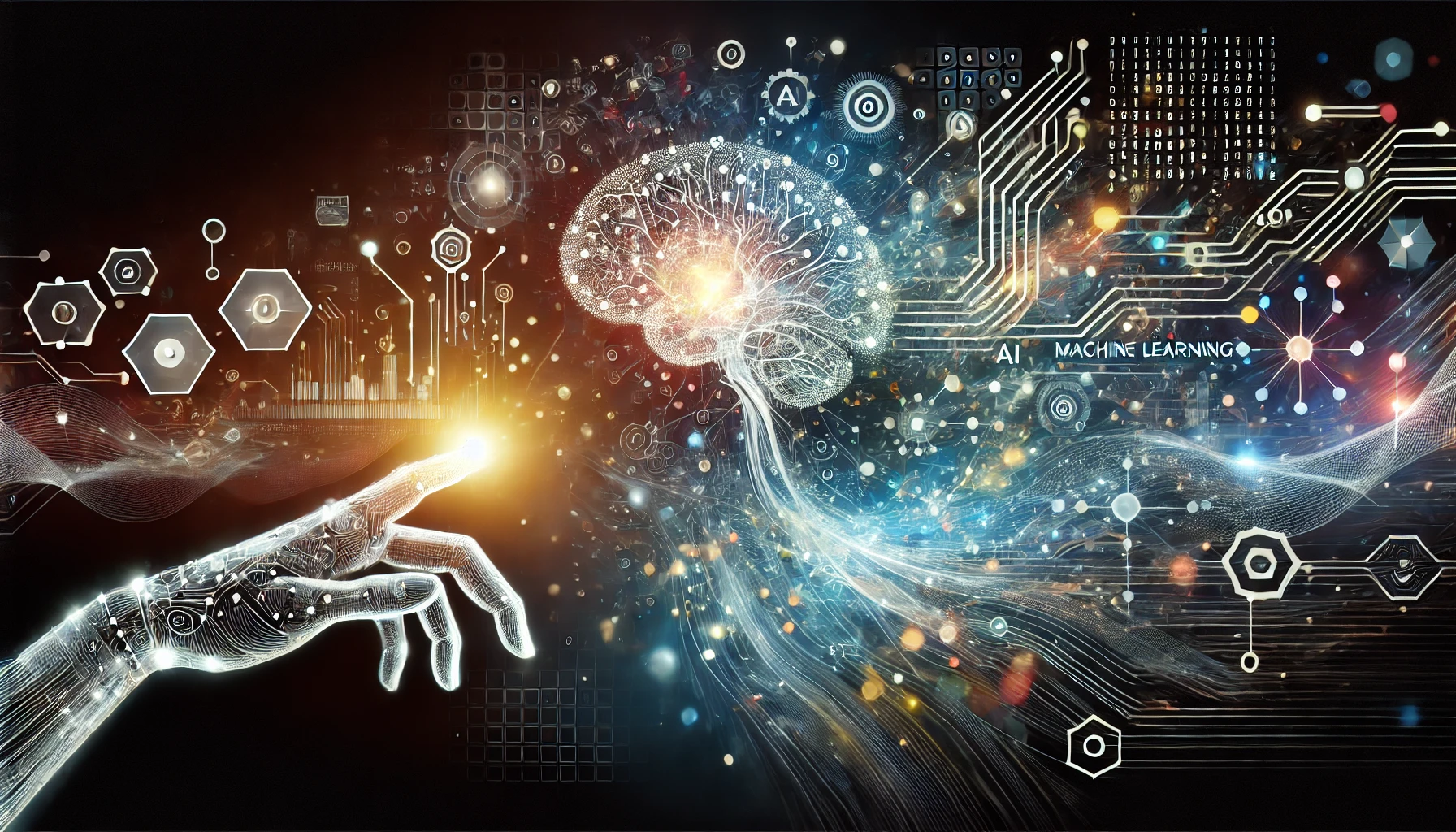
Chapter 1. Introduction
1.1. Understanding the Current State of AI
Artificial Intelligence (AI) has undergone a remarkable transformation over the past decade, becoming a cornerstone of technological advancement. Pioneering companies like OpenAI and Anthropic have spearheaded these developments, introducing increasingly sophisticated models that have reshaped our understanding of AI’s capabilities. From the groundbreaking release of ChatGPT to the emergence of competitors like Claude, the field has experienced rapid growth and diversification. These advancements have revolutionized industries by enhancing natural language processing, machine learning, and predictive analytics. AI models are now capable of performing complex tasks with unprecedented accuracy and speed, from generating human-like text to diagnosing diseases. As a result, AI has become an integral part of various sectors, including healthcare, finance, and entertainment, driving efficiency and innovation[1].
1.2. The Concept of an AI Plateau
Despite these impressive achievements, there is a growing discourse around the possibility of an AI plateau. This concept suggests that the pace of AI development may be slowing, with diminishing returns on investment in further advancements. This perception is fueled by several factors, including the inherent complexity of developing more sophisticated models and the physical limitations of current computing architectures. The rapid initial advancements in AI may have set high expectations for continuous progress, but as the field matures, achieving significant breakthroughs becomes more challenging. The complexity of AI models, which require massive amounts of data and computational power, adds to the difficulty of maintaining the same trajectory of innovation. Additionally, the reliance on established architectures, such as GPUs, may limit the scope for further transformative changes without new technological breakthroughs[2].
1.3. Purpose of the Article
This article aims to explore the diverse opinions surrounding the notion of an AI plateau. By examining various perspectives, we will delve into the technological, economic, and environmental factors that contribute to this debate. Through a critical analysis of the current state of AI and potential future directions, this article seeks to provide a comprehensive understanding of the challenges and opportunities that lie ahead. Key areas of focus will include the role of emerging technologies, the impact of market dynamics, and the potential for new architectures to redefine the landscape of AI. By addressing these topics, the article will offer insights into the future of AI development and its implications for industries and society at large.
Chapter 2. Technological Factors Influencing AI Progress
2.1. The Role of Moore’s Law in AI Development
Moore’s Law, coined by Intel co-founder Gordon Moore in 1965, predicted that the number of transistors on a microchip would double approximately every two years, resulting in exponential growth in computing power. This prediction held true for decades, driving the rapid evolution of technology and enabling the sophisticated AI systems we see today. However, as we approach the physical limitations of chip manufacturing, the continuation of Moore’s Law is increasingly in question.
Historical Significance
- Technological Growth: Moore’s Law has been a cornerstone of technological growth, propelling innovations across computing, telecommunications, and consumer electronics.
- Impact on AI: The law has enabled AI models to grow in complexity and capability, allowing for the development of advanced machine learning algorithms and neural networks.
Current Challenges
- Physical Limits: As transistors become smaller, approaching atomic scales, issues such as heat dissipation and quantum tunneling arise, complicating further miniaturization.
- Manufacturing Complexity: The intricacies of producing smaller chips have escalated manufacturing costs and reduced the number of companies capable of producing cutting-edge technology[2].
2.2. Computational Limits and Diminishing Returns
As AI models continue to grow in size and complexity, the returns on increased computational power are beginning to diminish. This phenomenon raises concerns about the sustainability of current AI development practices.
Computational Challenges
- Power Requirements: Larger AI models require immense computational resources, leading to increased energy consumption and costs.
- Performance Gains: While computational power has traditionally led to better AI performance, recent improvements are becoming less significant compared to the resources invested.
Examples of Diminishing Returns
- Language Models: The transition from GPT-3 to GPT-4 saw less dramatic improvements in language understanding and generation capabilities than previous iterations.
- Vision Systems: In image recognition tasks, doubling the size of datasets or model parameters yields smaller accuracy gains compared to initial increases[2].
2.3. Emerging AI Architectures and Innovations
In response to the limitations of traditional computing architectures, new approaches are being explored to sustain AI’s growth trajectory. These innovations have the potential to reshape the landscape of AI development.
New Architectural Approaches
- Analog AI Chips: Companies like IBM are investigating analog AI chips, which mimic the brain’s neural processing, potentially offering significant energy efficiency gains.
- Neuromorphic Computing: This approach seeks to design hardware that emulates the brain’s architecture, promising faster and more efficient processing for AI tasks.
Opinions on Overcoming Limitations
- Optimistic Views: Proponents believe these emerging technologies could bypass current constraints, enabling continued AI advancement without relying solely on traditional scaling.
- Skeptical Perspectives: Critics argue that while promising, these innovations face significant technical and commercial hurdles before they can meaningfully impact AI development[2].
In summary, while technological factors like Moore’s Law and computational limits present challenges to AI progress, emerging innovations offer hope for overcoming these barriers. The future of AI development may hinge on our ability to harness these new architectures and adapt to the changing technological landscape.
Chapter 3. Economic and Market Dynamics
3.1. Resource Allocation and Costs
The development of advanced AI models involves significant financial investment, with costs spanning research, infrastructure, and talent acquisition. As AI systems become more complex, the resources required to support them grow exponentially.
Financial Implications
- Research and Development Costs: Companies must invest heavily in R&D to remain competitive, which includes acquiring cutting-edge technology and hiring top-tier talent.
- Infrastructure Investment: The need for specialized hardware, such as GPUs and TPUs, drives up infrastructure costs. Maintaining these systems requires substantial capital, affecting the bottom line of AI enterprises.
Environmental Costs
- Energy Consumption: Large-scale AI models consume vast amounts of energy, contributing to their environmental footprint. The electricity required to train these models is substantial, leading to increased carbon emissions.
- Sustainability Concerns: As awareness of environmental impacts grows, companies face pressure to adopt sustainable practices, balancing innovation with ecological responsibility[2].
3.2. Market Competition and AI Commoditization
The AI landscape is characterized by intense competition, with numerous players vying for dominance. This competitive environment drives rapid innovation but also leads to the commoditization of AI technologies.
Impact on Development and Innovation
- Pressure to Innovate: Companies must continually innovate to maintain a competitive edge, leading to faster cycles of product development and deployment.
- Standardization: As AI technologies become more widespread, standardization occurs, resulting in similar performance across different models and diminishing unique competitive advantages.
Trend Toward Commoditization
- Interchangeability: AI solutions are increasingly seen as interchangeable, with businesses selecting tools based on cost-effectiveness rather than distinct capabilities.
- Pricing Pressures: The commoditization of AI leads to pricing pressures, forcing companies to find new ways to differentiate their offerings and capture market share[2].
3.3. Impact of Big Tech Dominance
The dominance of major technology companies in AI research and development presents both opportunities and challenges for the industry. These tech giants wield significant influence over the direction of AI progress.
Role in AI Development
- Research Leadership: Companies like Google, Microsoft, and Amazon lead in AI research, leveraging vast resources to push the boundaries of what’s possible.
- Investment in Innovation: Their financial power enables substantial investment in new technologies and talent, driving forward the field of AI.
Debate on Concentration of Power
- Benefits of Dominance: Proponents argue that big tech’s resources and expertise accelerate innovation and bring cutting-edge solutions to market quickly.
- Drawbacks of Concentration: Critics contend that this concentration of power stifles competition, limits diversity in research approaches, and creates barriers for smaller companies attempting to enter the market[2].
In examining these economic and market dynamics, it becomes clear that while AI presents vast opportunities for growth and innovation, it also poses significant challenges. Balancing resource allocation, market competition, and the influence of major players will be crucial in shaping the future landscape of AI development.
Chapter 4. Innovations and Future Directions
4.1. Specialized AI Systems and Narrow AI
Specialized AI systems, often referred to as narrow AI, are designed to perform specific tasks exceptionally well. Unlike general AI, which aims to mimic human intelligence across a broad range of activities, narrow AI focuses on optimizing performance in defined areas.
Advantages of Specialized AI
- Efficiency: Narrow AI systems are tailored to specific tasks, allowing them to operate with high efficiency and accuracy. For instance, AI in medical imaging can detect anomalies in scans faster and more accurately than human practitioners.
- Cost-Effectiveness: By concentrating on a single domain, specialized AI requires fewer resources to develop and maintain compared to broader AI systems, making it a cost-effective solution for businesses.
Potential for Industry Advancements
- Healthcare: AI systems are revolutionizing diagnostics, treatment planning, and patient monitoring, leading to improved patient outcomes and streamlined operations.
- Finance: Narrow AI is employed in fraud detection, algorithmic trading, and risk assessment, providing financial institutions with powerful tools to enhance security and efficiency.
- Manufacturing: AI-driven automation in manufacturing optimizes production lines, reduces waste, and enhances quality control, boosting productivity[2].
4.2. The Importance of Algorithmic Innovation
Algorithmic innovation is at the heart of AI progress, enabling breakthroughs that enhance the capabilities and efficiency of AI models. Developing new algorithms or improving existing ones can significantly impact AI performance and applicability.
Role in AI Progress
- Optimization: Innovative algorithms optimize data processing and model training, reducing computational requirements and improving speed. For example, advancements in gradient descent techniques have accelerated the training of deep learning models.
- Adaptability: Algorithms that enhance the adaptability of AI systems allow them to learn from smaller datasets, increasing their utility in environments with limited data availability.
Examples of Algorithmic Breakthroughs
- Transformer Models: The introduction of transformer architectures has revolutionized natural language processing, enabling models like GPT-3 to understand and generate human-like text.
- Reinforcement Learning: Innovations in reinforcement learning algorithms have led to AI systems capable of mastering complex games and simulations, demonstrating their potential in real-world applications[2].
4.3. Hybrid AI Models and Human-AI Collaboration
Hybrid AI models combine the strengths of AI systems with human expertise, creating collaborative environments where both can excel. This approach leverages the precision of AI with the creativity and contextual understanding of humans.
Benefits of Hybrid Models
- Enhanced Decision-Making: Hybrid models provide decision-makers with AI-generated insights while allowing human oversight, leading to more balanced and informed outcomes.
- Increased Efficiency: By automating routine tasks, AI frees up human experts to focus on strategic and creative aspects of their work, boosting overall productivity.
Applications in Various Sectors
- Healthcare: Hybrid systems assist doctors by analyzing patient data and suggesting treatment options, while the final decisions remain with healthcare professionals.
- Creative Industries: AI tools support artists, designers, and writers by generating ideas and drafts, enabling creative professionals to refine and perfect their work[2].
Innovations in specialized AI systems, algorithmic advancements, and hybrid models are paving the way for a future where AI continues to transform industries and society. By focusing on these areas, we can harness the full potential of AI to drive progress and create new opportunities.
Chapter 5. Conclusion
5.1. Summarizing Key Opinions
The debate over whether artificial intelligence is reaching a plateau encompasses a wide range of perspectives. Some experts argue that AI development is indeed slowing due to physical and computational limitations, as well as the saturation of certain markets. They point to diminishing returns in model performance and the challenges in developing new architectures that can sustain past growth rates. Conversely, others believe that AI still holds vast potential for innovation, particularly through the exploration of specialized applications, novel algorithms, and hybrid models that combine human and machine intelligence[2].
5.2. Looking Ahead: The Future of AI Innovation
The path forward for AI will likely require a multifaceted approach. Continued investment in emerging technologies and the exploration of unconventional architectures, such as neuromorphic and quantum computing, could unlock new levels of performance. Additionally, fostering collaboration between industry leaders, academic institutions, and governments will be crucial in addressing the economic and environmental challenges associated with AI development. By focusing on sustainability and ethical considerations, the AI community can ensure that technological progress benefits society as a whole.
Potential Paths Forward
- Interdisciplinary Collaboration: Bringing together experts from diverse fields to tackle complex AI challenges and promote innovation.
- Sustainability Initiatives: Prioritizing the development of energy-efficient AI systems to reduce environmental impact and promote long-term growth.
- Ethical AI Practices: Implementing frameworks that ensure AI technologies are developed and deployed in ways that are fair, transparent, and aligned with societal values[2].
5.3. Final Thoughts
Artificial intelligence remains a dynamic and rapidly evolving field with immense potential to transform industries and improve quality of life. While there are legitimate concerns about the sustainability and scalability of current AI models, ongoing research and innovation offer promising avenues for continued advancement. By embracing a forward-thinking mindset and remaining open to new ideas and technologies, the AI community can overcome current limitations and pave the way for a future of endless possibilities. Encouraging open dialogue and collaboration across sectors will be key to realizing the full potential of AI and ensuring its positive impact on the world.
Frequently Asked Questions (FAQs)
What is the current state of AI development?
The current state of AI development is marked by both rapid advancements and emerging challenges. Leading companies such as OpenAI and Anthropic have introduced cutting-edge models like ChatGPT and Claude, which showcase significant improvements in natural language processing and machine learning capabilities. These advancements have propelled AI into mainstream applications across industries, from healthcare and finance to entertainment and education. However, there is a growing perception that AI development might be reaching a plateau. This stems from several factors, including the increasing complexity of AI models, the immense computational resources required, and the diminishing returns on investment in terms of performance gains. Despite these challenges, the potential for innovation remains strong, with ongoing research exploring new architectures, such as neuromorphic computing and quantum AI, which could drive future growth[2].
How does Moore’s Law relate to AI progress?
Moore’s Law, a principle that has guided the semiconductor industry for decades, states that the number of transistors on a microchip doubles approximately every two years, leading to exponential increases in computing power. This law has played a crucial role in the evolution of AI, as more powerful processors have enabled the development of complex AI models capable of processing vast amounts of data. However, the limitations of Moore’s Law are becoming increasingly apparent as we approach the physical boundaries of chip miniaturization. This has implications for AI progress, as the rate of hardware improvement slows, potentially constraining the growth of AI capabilities. To address this, researchers and engineers are exploring alternative computing paradigms that could bypass these limitations and sustain AI advancement[2].
What are the economic implications of AI development?
The economic implications of AI development are profound, influencing industries, labor markets, and global economies. On one hand, AI technologies have the potential to drive significant productivity gains, enhance operational efficiency, and create new markets and opportunities. For businesses, this translates into improved competitiveness and the potential for cost savings and revenue growth. Conversely, the rise of AI also poses challenges, particularly in terms of job displacement and economic inequality. As AI systems automate routine tasks, certain jobs may become obsolete, necessitating workforce reskilling and adaptation. Additionally, the concentration of AI research and development within major tech companies raises concerns about market dominance and the equitable distribution of AI’s benefits. Addressing these challenges requires a balanced approach that fosters innovation while ensuring economic inclusivity and fairness[2].
What innovations could drive future AI growth?
Several innovations hold the potential to drive future AI growth and overcome current limitations. Key areas of focus include:
- Neuromorphic Computing: Inspired by the human brain’s architecture, neuromorphic computing seeks to develop hardware that mimics neural networks, offering the potential for more efficient and adaptive AI systems.
- Quantum AI: Leveraging the principles of quantum mechanics, quantum AI could revolutionize data processing and problem-solving, enabling AI models to tackle complex tasks beyond the reach of classical computing.
- Hybrid AI Models: By combining AI with human expertise, hybrid models can enhance decision-making and creativity, creating systems that leverage the strengths of both artificial and human intelligence.
- Algorithmic Breakthroughs: Continued advancements in algorithms, such as improved machine learning techniques and optimization methods, can enhance AI performance and broaden its applicability across diverse domains.
These innovations represent exciting avenues for exploration and investment, promising to push the boundaries of what AI can achieve and transform the technological landscape[2].
Citations:
[1] https://spectrum.ieee.org/deep-learning-computational-cost
[2] https://www.linkedin.com/pulse/differences-between-gpt-3-gpt-4-progress-ai-language-models-speakt
[3] https://www.nature.com/articles/s41586-023-06337-5
[4] https://p4sc4l.substack.com/p/ai-about-the-idea-that-ai-development
[5] https://community.openai.com/t/being-charged-for-gpt-4-but-getting-gpt-3/446228
[6] https://research.ibm.com/blog/analog-ai-chip-low-power
[7] https://www.linkedin.com/pulse/diminishing-returns-ai-aragorn-meulendijks-mhvfe